Search platforms (that rely on different business models) such as Amazon, Google or Yelp possess an abundance of consumer-specific data from past searches, purchases, and the behavior of ‘similar’ consumers. When a consumer searches for a product (or service) using a platform’s interface, the platform can invoke this knowledge when listing search results. In creating this list, it has become customary for platforms to sell prominently featured slots, so-called sponsored slots or positions, to firms hoping to steer consumers towards buying their products. Google founders Sergey Brin and Larry Page were on record, as early as 1998, warning of the implications of search advertising: “We expect that advertising funded search engines will be inherently biased towards the advertisers and away from the need of the consumers.” In fact, some of today’s consumers are reluctant to click on sponsored search results to circumvent steering by firms, as they believe non-sponsored (organic) search results promise to be a better match for their preferences.
In “Search Platforms: Big Data and Sponsored Positions,” co-authored by Maarten Janssen, Thomas Jungbauer, Marcel Preuss and Cole Williams, we argue that the issue at hand is more complicated. In fact, we introduce a game-theoretic model analyzing the interaction between a search platform, consumers and firms to show that, when the number of firms is large, it is in the best interest of the consumer to click on sponsored positions, while the introduction of sponsored positions in the first place may either benefit or harm consumers, depending on the platform’s competing objectives. For example, if the platform (also) maximizes sales commission revenue—as is common practice—sponsored slots are beneficial for the consumer. This is because platforms face an incentive to allocate sponsored positions to firms that constitute good matches for consumers in order to increase the revenue from auctions for sponsored positions. On the other hand, obfuscation of organic slots plays a big role in optimal search platform behavior. First, it reduces the consumer’s option value from searching beyond sponsored positions, and, second, it increases the number of positions a consumer inspects if they continue to search among organic slots, thereby increasing the probability of a sale being made.
Our results persist independently of the allocation of consumer-specific knowledge between the search platforms and firms and the variation in prices among firms. Among the major complications to overcome when characterizing optimal platform behavior is that consumers, when inspecting a firm in a given slot, learn about firms in slots they have not yet inspected when they understand the search platform’s algorithm. As a result, we can show that in environments with few firms only, the optimal algorithm of the search platform always depends on the intricacies of the specific problem, and no general rule for the ranking of search results can exist. However, when the number of firms is large—as is customary when dealing with search platforms—the platform behavior as described above is optimal almost surely. In order to prove our results with many firms, we adapt a result from the literature on social learning, the so-called mixing property of stochastic processes, for consumer search problems.
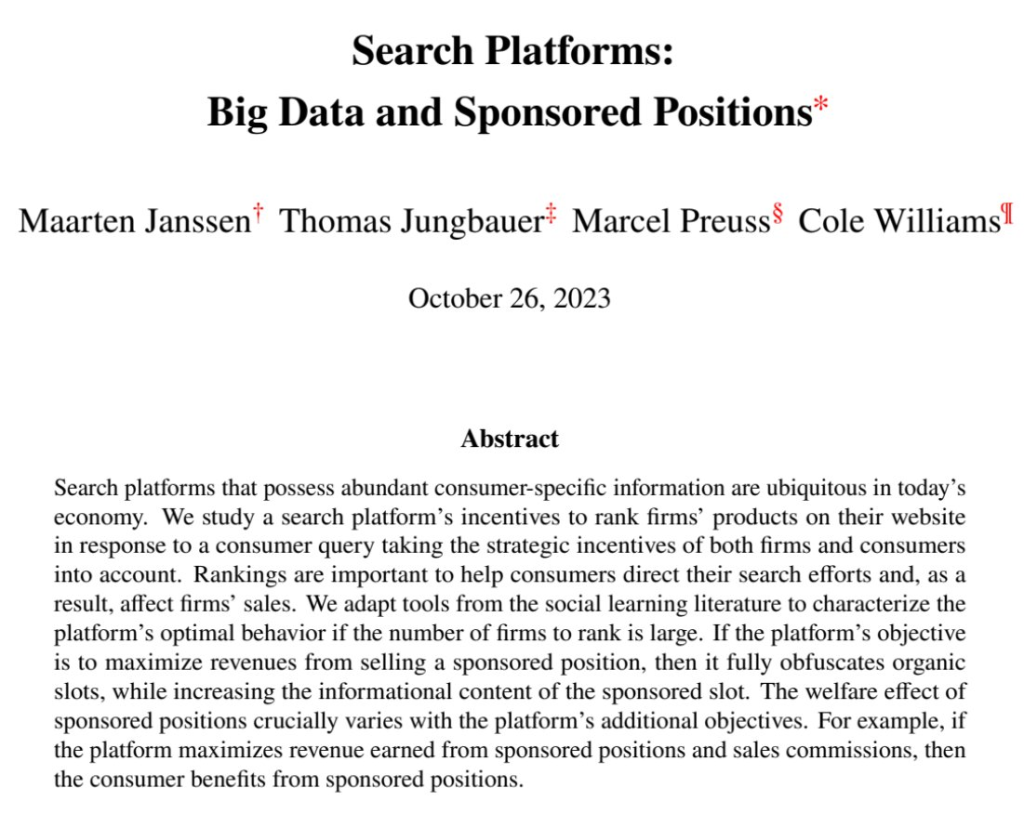